Author: Mathangi Gopalakrishnan, MPharm, PhD, Shamir Kalaria, PharmD, PhD, and Rahul Goyal on December 01, 2022 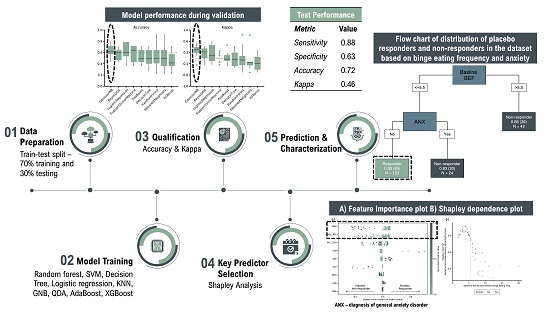
Placebo responders, defined as subjects experiencing a clinically meaningful reduction in disease symptomology when randomized to receive placebo, is one of the major reasons of trial failure in binge eating disorder (BED) trials. Among investigator-led, randomized, placebo-controlled trials evaluating patients with BED, 50% of trials demonstrated a lack of a statistically significant separation from placebo. Enriching clinical trials by excluding placebo responders at baseline and prior to entering the double-blind period can help increase probability of BED trial success. Supervised machine learning (ML) algorithms are a set of tools that can guide model development by mapping inputs and outputs without having to explicitly program them. Given that ML can model complex non-linear relationships, continually improve, and handle large amounts of data, their usage in drug development has increased over the past decade. Through our study, we aimed to build supervised ML models to identify moderators of placebo response in patients with BED for the purpose of future trial enrichment.
Different baseline variables – current and historical diagnosis of comorbid psychiatric conditions, BED severity, and patient demographics – were used as inputs to classify a dataset of 189 subjects as placebo responder or placebo non-responder. The dataset was pooled from 12 similar investigator-led prospective clinical trials. Placebo response was defined as greater than 75% reduction in disease severity at study endpoint. Algorithms tested as part of model training (using grid search on 10-fold cross validation) were random forest, support vector machines, decision tree, logistic regression, k-nearest neighbors, Gaussian naïve Bayes (GNB), Bayesian quadratic discriminant analysis, AdaBoost, and XGBoost.
The GNB algorithm demonstrated the best mean validation performance (accuracy of 64% and Cohen’s Kappa of 0.31). On the testing dataset, the GNB model showcased 88% sensitivity and 72% accuracy which signified adequate prediction performance on unseen data. Baseline BED severity and a co-morbid diagnosis of general anxiety disorder were identified as the top two predictors of placebo response. Upon further characterization of the interaction between these two variables using Shapley analysis, we found that patients with a baseline BED severity of less than 5.5 episodes per week and no anxiety were more likely to be placebo responders as compared to others. The presented study results1 should be validated in future studies so that the application of these findings can be used to inform the inclusion/exclusion criteria of BED trials.

The comment feature is locked by administrator.