Author: Deanna L. Kroetz, PhD on December 10, 2018 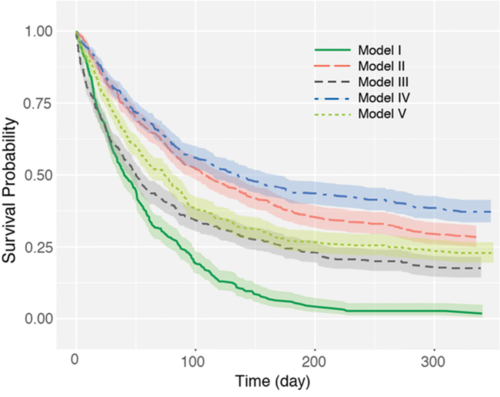
Generating “big data” has become increasingly easier and less expensive over the last decade, and the need for computational methods for the analysis of these data are fueling the burgeoning field of data sciences. The challenge for clinical pharmacology will be to rapidly assess and apply new computational methods to complex drug response data.
Survival models are often used to define drug efficacy, especially in the field of oncology. These data are typically analyzed using a Cox proportional hazard regression model, even when the assumptions that the hazard function of predictor variables are constant over time and additive are known or suspected to be violated. A recent article by Gong and colleagues in Clinical and Translational Science supports the application of machine learning for time-to-event analyses when the predictor variables assume nonlinear relationships. These investigators compared the results of two machine learning approaches, artificial neural networks and random survival forests, to the Cox proportional hazard regression method applied to simulated data with increasingly complex and nonlinear relationships. Their results highlight the risk of applying the Cox model to data that are not linear or additive for covariates in the hazard function. Either machine learning approach more accurately predicted increasingly complex relationships between the predictor variables than the Cox proportional hazard model. The authors also highlight an added benefit of machine learning approaches to time-to-event data that the methods are robust to high dimensional data. It will be interesting to apply the methods described in this paper to dose-dependent toxicities such as drug-induced peripheral neuropathies and other time-dependent drug phenotypes.
This is an exciting time to be involved in translational research and the field will benefit from the application of robust computational methods for data analysis. The Clinical and Translational Science editorial team invites the submission of manuscripts focused on the application of machine learning and related approaches to the analysis of complex drug and toxicity datasets.
Image by Gong et al. Clin. Trans. Sci., doi.org/10.1111/cts.12541, is licensed under CC BY-NC-ND 4.0. ©2018 The authors.

The comment feature is locked by administrator.