Author: Julia Wattacheril, MD, MPH, and Anna O. Basile, PhD on March 25, 2025 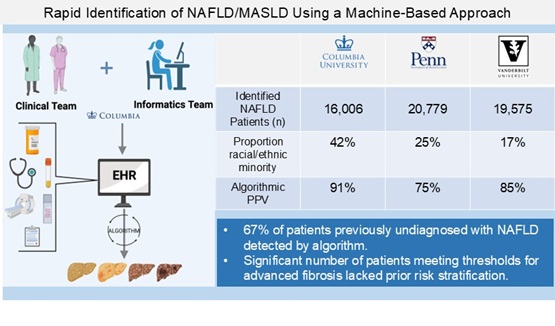
Identification and stratification of patients with under-recognized metabolic disease is critical in addressing the Nonalcoholic Fatty Liver Disease (NAFLD)* public health crisis. Reliance on frontline healthcare recognition alone may contribute to delays in treatment, monitoring, and referrals for specialty care, allowing earlier and further disease progression. Beyond clinician education and public awareness campaigns, additional scalable, automated, rapidly deployable tools are necessary for effective point-of-care intervention. Systemic improvements through electronic health record (EHR)-based methods may help streamline the identification of potential disease in patient populations, and with effective validation and linkage to care, may help deliver on the promise of more precise care. Healthcare systems with interrogatable longitudinal data provide means of discovering patients at risk for NAFLD progression and related disease manifestations.
Our work introduces an algorithm that successfully identifies NAFLD and Nonalcoholic Steatohepatitis (NASH) patients across three diverse healthcare systems (>12 million patients). Sixty-seven percent of the NAFLD patients discovered at Columbia University Irving Medical Center were previously undiagnosed by ICD-9/10 codes alone. Our machine-based approach first identifies patients at risk of NAFLD using diagnoses and risk indicators, then excludes patients with confounding diagnoses, and finally verifies hepatic steatosis. Institutions supporting the Observational Medical Outcomes Partnership (OMOP) Common Data Model (CDM) can implement this approach to identify at-risk patients for downstream clinical referrals and investigation, including rare diseases that may mimic NAFLD. Further stratification with noninvasive tests can identify and triage patients with advanced fibrosis at scale. Our rule-based algorithm represents the first stage of development in machine learning and AI-driven methods aimed at predicting, stratifying, and referring for intervention and linkages to care in NAFLD.
Immediate real-world applications in common complex metabolic diseases like NAFLD include rapid triage and subsequent clinical validation. The emergence of automated approaches augmenting complex care delivery has the potential to deliver precision care, alleviate provider time burdens and expertise mismatch, identify misdiagnoses, improve patient awareness of disease stage within the context of existing care and clinical trials, and empower patient-provider decisions regarding treatment modalities.
*nomenclature change to metabolic dysfunction associated steatotic liver disease (MASLD)/ metabolic dysfunction associated steatohepatitis (MASH) followed original development of algorithmic code

The comment feature is locked by administrator.